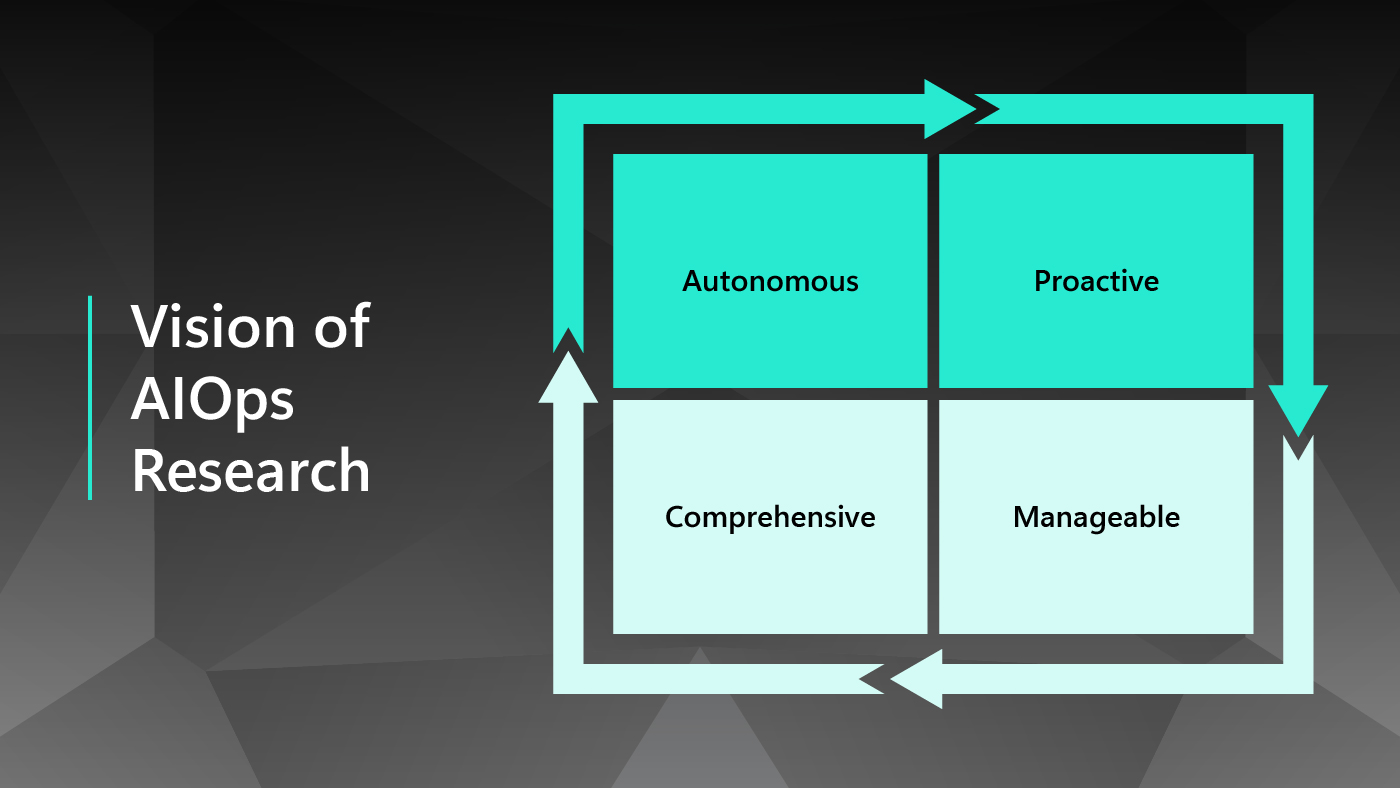
Cloud Intelligence/AIOps weblog website online sequence
In the first actual submit on this sequence, Cloud Intelligence/AIOps– Instilling AI into Cloud Computing Programs, we supplied a snappy abstract of Microsoft’s analysis learn about on Cloud Intelligence/AIOps (AIOps), which innovates AI and synthetic intelligence (ML) inventions to lend a hand taste, broaden, and run sophisticated cloud platforms and products and services successfully and successfully at scale. As cloud computing platforms have if truth be told persisted to develop into a few of the most simple amenities of our international, each their scale and intricacy have if truth be told grown considerably. In our earlier submit, we went over the three important pillars of AIOps analysis learn about: AI for Programs, AI for Shoppers, and AI for DevOps, at the side of the 4 important analysis learn about places that make up the AIOps factor space: detection, scientific prognosis, forecast, and optimization. We likewise visualized the AIOps analysis learn about roadmap as construction in opposition to generating extra self-governing, proactive, workable, and thorough cloud platforms.
Imaginative and prescient of AIOps Analysis Find out about
Self-governing | Proactive | Workable | Complete |
Utterly automate the operation of cloud techniques to lower device downtime and reduce handbook efforts. | Expect long run cloud standing, help proactive decision-making, and steer clear of unhealthy issues from happening. | Provide the idea that of tiered autonomy for instilling self-governing common operations and deep human skillability. | Duration AIOps to the entire cloud stack for international optimization/control and surround multi-cloud environments. |
Starting with this submit, we will be able to take a far deeper dive into Microsoft’s imaginative and prescient for AIOps analysis learn about and the continual efforts to acknowledge that imaginative and prescient. This submit will be aware of how our scientists leveraged complex AIOps analysis learn about to lend a hand make cloud inventions extra self-governing and proactive. We will be able to speak about our paintings to make the cloud extra workable and thorough in long run submit.
Self-governing cloud
Inspiration
Cloud platforms want quite a lot of movements and alternatives each second to make certain that calculating sources are accurately treated and screw ups are right away resolved. In apply, the ones movements and alternatives are both created by means of rule-based techniques constructed upon skilled working out or made by means of hand by means of an expert engineers. Nonetheless, as cloud platforms keep growing in each scale and intricacy, apparently that such choices will likely be insufficient for the long run cloud device. On one hand, stiff rule-based techniques, whilst being working out empowered, often come with considerable sorts of tips and wish common repairs for significantly better coverage and flexibility. Nonetheless, in apply, it’s often impractical to stay such techniques up to date as cloud techniques develop in each measurement and intricacy, and much more tricky to verify consistency and save you disputes in between all of the tips. Then again, engineering efforts are extraordinarily long, prone to errors, and hard to scale.
Highlight: On-Call for Instance
Microsoft Analysis Find out about Most sensible 2022
On-Call for
See now to find some of the maximum essential issues dealing with our analysis learn about community and pay attention to discussions with 120+ scientists round how to verify brand-new inventions have the broadest conceivable merit for humankind.
To damage the restraints at the coverage and scalability of the present choices and toughen the flexibility and manageability of the decision-making techniques, cloud platforms will have to transfer in opposition to a extra self-governing control paradigm. Relatively of depending solely on skilled working out, we require perfect AI/ML designs to fuse useful data {and professional} working out in combination to make it conceivable for efficient, unswerving, and self-governing control alternatives. Nonetheless, it’ll take a large number of analysis learn about and engineering efforts to eliminate other limitations for setting up and freeing self-governing choices to cloud platforms.
In opposition to a self-governing cloud
Within the adventure in opposition to a self-governing cloud, there are 2 important difficulties. The first actual impediment is determined by the heterogeneity of cloud data. In apply, cloud platforms free up a considerable number of shows to collect data in several codecs, consisting of telemetry alerts, machine-generated log information, and human enter from engineers and customers. And the patterns and circulations of the ones data generally display a top level of selection and go through changes in time. To make certain that the embraced AIOps choices can paintings autonomously in such an atmosphere, it is very important empower the control device with tough and extendable AI/ML designs environment friendly in finding useful main points from heterogeneous data resources and drawing perfect conclusions in several eventualities.
The sophisticated interplay in between quite a lot of portions and products and services supplies every other important impediment in freeing self-governing choices. Whilst it may be easy to execute self-governing purposes for one or a few parts/products and services, find out how to construct end-to-end techniques environment friendly in right away surfing the advanced dependences in cloud techniques supplies the true impediment for each scientists and engineers. To unravel this impediment, it is important to to profit from each area working out and data to toughen the automation lessons in utility eventualities. Scientists and engineers should likewise execute unswerving decision-making algorithms in each selection section to toughen the efficiency and steadiness of all the end-to-end decision-making process.
Over the last few years, Microsoft analysis learn about teams have if truth be told established a large number of brand-new designs and methods for conquering the ones difficulties and embellishing the extent of automation in several cloud utility eventualities during the AIOps factor spaces. Vital examples encompass:
- Detection: Gandalf and ATAD for the early detection of difficult implementations; HALO for hierarchical malfunctioning localization; and Onion for figuring out incident-indicating logs.
- Scientific Prognosis: SPINAL COLUMN and UniParser for log parsing; Reasoning and Warden for regression and incidence scientific prognosis; and CONAN for batch failure scientific prognosis.
- Forecast: TTMPred for forecasting time to relieve occurrences; LCS for forecasting the low-capacity standing in cloud servers; and Expulsion Forecast for forecasting the expulsion of space digital makers.
- Optimization: MLPS for reinforcing the reallocation of bins; and RESIN for the control of reminiscence leakage in cloud amenities.
Those choices no longer simply toughen provider efficiency and reduce control time with extra automatous taste, alternatively likewise result in larger potency and dependability with much less human errors. For instance of our pursue a extra self-governing cloud, we will be able to speak about our expedition for supporting computerized secure implementation products and services indexed beneath.
Superb state of affairs: Computerized secure implementation
In on-line products and services, the consistent mixture and dependable implementation (CI/CD) of brand-new spots and builds are important for the suggested cargo of trojan horse maintenance and serve as updates. Since brand-new implementations with undiscovered insects or incompatible issues can cause excessive provider disasters and broaden considerable consumer impact, cloud platforms put in force rigorous safe-deployment remedies previous to launching every brand-new implementation to the manufacturing environments. Such remedies generally come with multi-stage screening and affirmation in a chain of canary environments with expanding scopes. When a deployment-related abnormality is decided in amongst those levels, the responsible implementation is rolled again for extra scientific prognosis and repairing. Owing to the difficulties of figuring out deployment-related abnormalities with heterogeneous patterns and dealing with a considerable number of implementations, safe-deployment techniques supervised by means of hand may also be very dear and blunder inclined.
To toughen computerized and constant anomaly detection in secure implementation, we proposed a fundamental manner known as ATAD for the environment friendly detection of deployment-related abnormalities in time-series alerts. This manner offers with the difficulties of recording changes with other patterns in time-series alerts and the absence of recognized anomaly samples because of the heavy expense of labeling. Specifically, this manner integrates ideas from each switch understanding and lively understanding to make nice utilization of the temporal main points within the enter sign and reduce the number of recognized samples wanted for design coaching. Our experiments have if truth be told published that ATAD can surpass different complex abnormality detection tactics, even with simply 1% -5% of recognized data.
At the very same time, we labored along with merchandise teams in Azure to ascertain and free up Gandalf, an end-to-end computerized secure implementation device that lowers implementation time and will increase the precision of figuring out unhealthy implementation in Azure. As a data-driven device, Gandalf assists in keeping monitor of a large collection of main points, consisting of potency metrics, failure alerts and implementation information. It likewise identifies abnormalities in several patterns during the entire safe-deployment process. After figuring out abnormalities, Gandalf makes use of a vote-veto device to dependably determine whether or not every came upon abnormality is prompted by means of a specific brand-new implementation. Gandalf then right away chooses whether or not the fitting brand-new implementation should be picked up a restore or if it is secure sufficient to proceed to the following section. After presenting in Azure, Gandalf has if truth be told labored at helping to document unhealthy implementations, carrying out greater than 90% accuracy and close to 100% recall in manufacturing over a period of 18 months.

Proactive cloud
Inspiration
Same old decision-making within the cloud concentrates on bettering rapid useful resource use and resolving rising issues. Whilst this reactive taste isn’t unreasonable in a rather mounted device, it could possibly purpose short-sighted alternatives in a colourful atmosphere. In cloud platforms, each the will and utilization of calculating sources are going thru steady changes, consisting of regimen periodical patterns, unanticipated spikes, and modern shifts in each temporal and spatial measurements. To toughen the iconic efficiency and dependability of cloud platforms, it will be significant to embody a proactive taste that takes the long run standing of the device under consideration within the decision-making process.
A proactive taste leverages data-driven designs to look ahead to the long run standing of cloud platforms and make it conceivable for downstream proactive decision-making. Conceptually, a commonplace proactive decision-making device contains 2 modules: a forecast module and a decision-making module, as proven within the following diagram.

Within the forecast module, ancient data are collected and processed for coaching and tweak the forecast design for implementation. The launched forecast design takes within the on-line data circulation and produces forecast result in precise time. Within the decision-making module, each the prevailing device standing and the forecasted device standing, along with different main points reminiscent of area working out and former selection historical past, is thought of for making alternatives that stabilize each provide and long run benefits.
In opposition to proactive taste
Proactive taste, whilst generating brand-new possibilities for reinforcing the iconic efficiency and dependability of cloud techniques, does reveal the decision-making process to further risks. On one hand, because of the intrinsic randomness within the on a regular basis operation of cloud platforms, proactive alternatives are repeatedly subjected to the unpredictability risk from the stochastic facets in each operating techniques and the environments. Then again, the dependability of forecast designs contains every other layer of risks in making proactive alternatives. For this reason, to verify the potency of proactive taste, engineers will have to put techniques in location to wait to these risks.
To take care of unpredictability risk, engineers require to reformulate the decision-making in proactive taste to constitute the unpredictability facets. They may be able to often make the most of methodological buildings, reminiscent of forecast+ optimization and optimization beneath chance-constraints, to combine unpredictabilities into the objective purposes of optimization problems. Correctly designed ML/AL designs can likewise uncover unpredictability from data for reinforcing proactive alternatives as opposed to unpredictability facets. With regards to risks associated with the forecast design, modules for reinforcing data high quality, consisting of quality-aware serve as engineering, tough data imputation, and data rebalancing, ought for use to attenuate forecast errors. Engineers should likewise make consistent efforts to toughen and improve the effectiveness of forecast designs. Moreover, securing techniques are essential to steer clear of alternatives that may cause injury to the cloud device.
Microsoft’s AIOps analysis learn about has if truth be told originated the shift from reactive decision-making to proactive decision-making, in particular in factor spaces of forecast and optimization. Our efforts no longer simply purpose considerable enhancement in a large number of utility eventualities usually supported by means of reactive decision-making, alternatively likewise broaden a large number of brand-new possibilities. Vital proactive taste choices encompass Narya and Nenya for {hardware} failure mitigation, UAHS and CAHS for the sensible digital software provisioning, CUC for the predictive scheduling of labor, and UCaC for bin packaging optimization beneath alternative restraints. Within the dialog indexed beneath, we will be able to make the most of {hardware} failure mitigation for example to turn how proactive taste can be utilized in cloud eventualities.
Superb state of affairs: Proactive {hardware} failure mitigation
An very important chance to cloud platforms is {hardware} failure, which is able to cause disruptions to the hosted products and services and significantly impact the customer revel in. Most often, {hardware} disasters are simply mounted reactively after the failure takes position, which generally contains temporal disruptions of hosted digital makers and the restore paintings or alternative of affected {hardware}. Any such provider provides minimum help in decreasing damaging consumer studies.
Narya is a proactive disk-failure mitigation provider environment friendly in taking mitigation movements previous to disasters occur. Specifically, Narya leverages ML designs to look ahead to conceivable disk disasters, and after that make alternatives correctly. To regulate risks associated with unpredictability, Narya assesses prospect mitigation movements founded upon the approximated results to purchasers and alternatives movements with minimal impact. A comments loop likewise exists for collecting follow-up reviews to toughen forecast and selection modules.
{Hardware} disasters in cloud techniques are often extraordinarily synergistic. For this reason, to attenuate the impact of forecasts errors, Narya items an distinctive dependency-aware design to encode the reliance dating in between nodes to toughen the failure forecast design. Narya likewise executes an adaptive method that makes use of A/B screening and outlaw modeling to toughen the potential to approximate the consequences of movements. Quite a lot of securing techniques in quite a lot of levels of Narya are likewise in location to take away the possibility of making dangerous mitigation movements. Software of Narya in Azure’s manufacturing atmosphere has if truth be told diminished the node {hardware} disturbance fee for digital makers by means of greater than 26%.
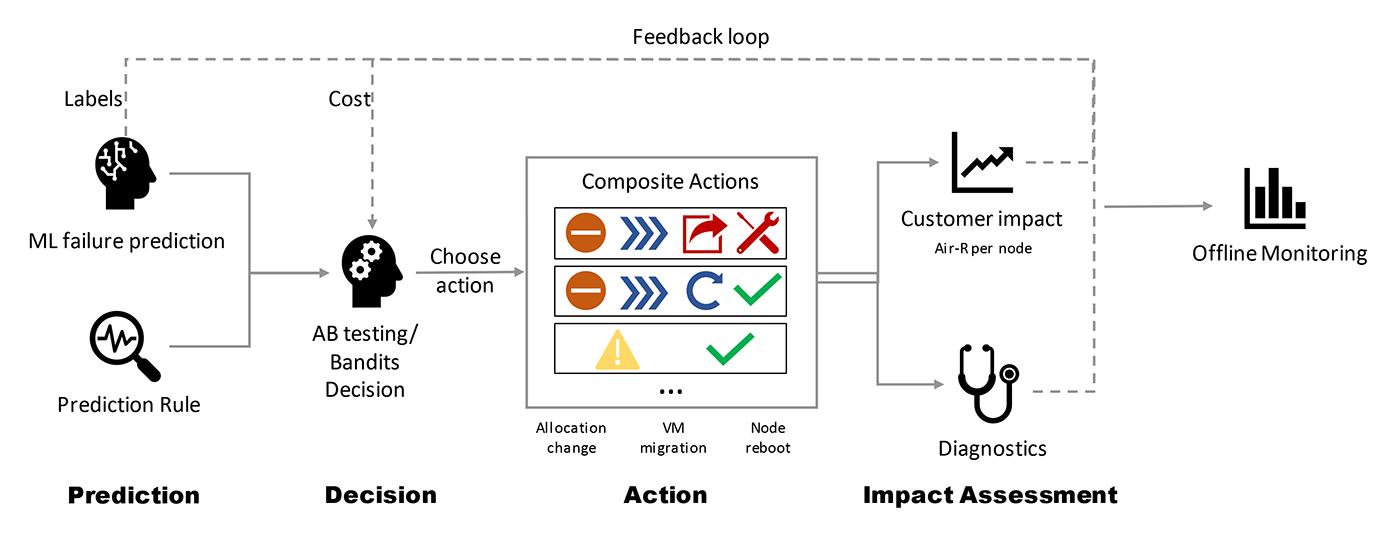
Our present paintings, Nenya, is every other instance for proactive failure mitigation. Underneath a toughen finding construction, Nenya merges forecast and decision-making modules into an end-to-end proactive decision-making device. It could possibly weigh each mitigation bills and failure charges to significantly better center of attention on economical mitigation movements as opposed to unpredictability. Moreover, the traditional failure mitigation manner usually studies data imbalance issues; circumstances of failure type only a in point of fact little a part of all circumstances, that have essentially wholesome situations. Such data imbalance would provide predisposition to each the forecast and decision-making process. To unravel this factor, Nenya embraces a cascading construction to make certain that mitigation alternatives aren’t made with heavy bills. Explores Microsoft 365 data units on database failure have if truth be told proven that Nenya can reduce each mitigation bills and database failure charges in comparison to current tactics.
Long run paintings
As control techniques finally end up being extra automated and proactive, it is important to to pay distinctive consideration to each the safety of cloud techniques and the duty to cloud purchasers. The self-governing and proactive selection device will rely very much on refined AI/ML designs with little handbook effort. Tips on how to make certain that the decisions made by means of the ones tactics are each secure and responsible is a vital worry that long run paintings ought to reply to.
The self-governing and proactive cloud is determined by the environment friendly data use and comments loop during all levels within the control and operation of cloud platforms. On one hand, top rate data at the standing of cloud techniques are required to make it conceivable for downstream self-governing and proactive decision-making techniques. Then again, it is important to to keep watch over and assessment the impact of every selection at the entire cloud platform with a purpose to toughen the control device. Such comments loops can exist suddenly for a large number of related utility eventualities. For this reason, to significantly better toughen a self-governing and proactive cloud, a blended data plane answerable for the processing and comments loop can take a primary serve as in all the device taste and should be an very important location of monetary funding.
As such, the way forward for cloud is based no longer simply on embracing extra self-governing and proactive choices, alternatively likewise on bettering the manageability of cloud techniques and the thorough infusion of AIOps inventions over all stacks of cloud techniques. In long run submit, we will be able to cross over find out how to pursue a extra workable and thorough cloud.
Keep tuned!